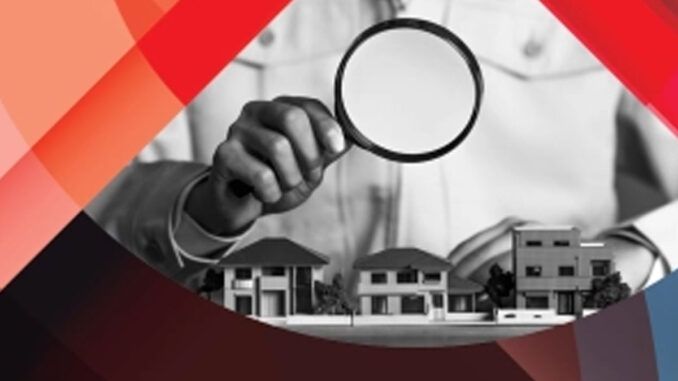
The National Association of Mortgage Brokers (NAMB) announced this week that it supports new federal regulatory proposals governing the use of automated valuation models (AVMs) in determining property values for mortgage originators and secondary market issuers.
Last month, federal regulators called for two changes to rules governing the use of AVMs: one that would require lenders to establish quality control standards for the creation of AVMs; and one which would add guidance regarding how and when appraisals can be challenged by banks and customers.
“The reality is the systems and structures are themselves, in some cases, problematic,” said NAMB President Ernest Jones in a statement. “Even when appraisers follow the intended approach, it may result in an outcome that disenfranchises people. They could be doing everything in a way they feel is consistent with the approaches they’ve learned and for which they’re certified, but there are some underlying issues that need to be addressed.”
Tackling appraisal bias has been a core concern of federal housing authorities during the Biden administration. When these AVM proposals were announced in June in part by Vice President Kamala Harris, she described the importance of tackling such changes by saying that “systemic change is needed.”
Last month, six federal agencies introduced a proposed rule that would implement quality control standards that govern the use of AVMs used by mortgage originators and secondary market issuers in valuing real estate collateral securing mortgage loans.
The agencies involved included the Federal Housing Finance Agency; the Consumer Financial Protection Bureau; the National Credit Union Administration; the Federal Deposit Insurance Corporation; the U.S. Department of the Treasury; and the Federal Reserve System.
In a blog post released with the joint announcement in June, CFPB Director Rohit Chopra said AVMs have the potential to cause serious harm if the algorithms are inaccurate or biased.
“While machines crunching numbers might seem capable of taking human bias out of the equation, they can’t,” Chopra said. “Based on the data they are fed and the algorithms they use, automated models can embed the very human bias they are meant to correct. And the design and development of the models and algorithms can reflect the biases and blind spots of the developers.”