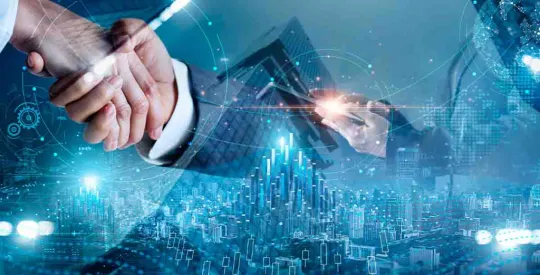
Artificial intelligence (AI) and machine learning (ML) present incredible opportunities to expand homeownership and housing in the U.S. But for potential homebuyers to benefit from new technology, the industry must figure out a way to keep bias out of decision-making models, experts said Monday.
“The biggest opportunity in my mind in the near to medium term remains [finding] ways to take unstructured data and leveraging that and turning it into machine readable machine information,” said Steve Holden, senior vice president of single-family analytics & modeling at Fannie Mae.
Holden spoke on the topic of leveraging data science and alternative data to increase access to homeownership at Mortgage Bankers Association’s (MBA’s) Technology Solutions Conference & Expo at San Jose, California on Monday.
Fannie Mae’s focus on incorporating machine learning is to get a rich profile of consumers’ risk and use that to evaluate the suitability for success in homeownership.
In particular, Holden noted the opportunity of training computer algorithms to review bank statements and identifying borrowers who have no credit but are making consistent rent payments every month.
“We should consider that (consistent rent payments) in their (borrowers’) evaluation of risk (…) If you’re a renter, none of these payments are considered. So this idea that you can go to the bank statement and extract that data and leverage it from the decision-making is a really powerful and important innovation to access certain sectors of the population,” Holden said.
Rocket Companies is focused on using technology to tap into a potential customer base.
Brian Stucky, lead for Rocket Ethical AI at Rocket Central, noted the rapidly growing Hispanic homeownership rate. By 2040, 70% of new homeowners will be Latino but many could be denied a mortgage because of their high debt-to-income levels.
“If Hispanics are falling out a lot of the time because of the DTI, what does that mean?(…) What is a part of that that we can potentially look at to make a little bit better because ultimately it’s going to be expanding underwriting in some fashion to include these aspects that might help us identify clients that are getting turned down now but are likely going to be good credit risks for us,” Stucky said.
From the housing supply side, machine learning models could help address bias in the collateral valuation process, Peter Carroll, executive of public policy & industry relations at CoreLogic, said.
“I’ve seen whole construction projects at their infancy stage (…) stopped dead in their tracks because there’s a perception that there aren’t going to be any sales comparable properties in the neighborhood and therefore there won’t be any mortgage financing, which is their (builders’) takeout and therefore there’s no point doing the project,” Carroll said.
America faces an undersupply of housing of 1.1 million units and growing with respect to single-family residential one- to four- unit entry level homes, according to CoreLogic.
There is a tremendous appetite from states, housing finance agencies, local subsidy divisions of cities and counties and zoning divisions to engage in this dialogue, Caroll said, noting the opportunities of technology in regards to addressing low housing supply.
The housing industry will need to figure out how to incorporate artificial intelligence and machine learning while keeping bias from creeping into the business decision-making model, he said.
“You’ve probably heard machine learning referred to as a black box, it is difficult to look at and understand why a decision was rendered from the inputs that it was given. That’s something that will have to be overcome before we have wholesale adoption and use across the industry,” Stucky explained.
Explaining the reasoning behind decisions coming out of the models will be important in a heavily regulated industry like housing, Holden said.
“One of the things we think about a lot is when we’re getting decisions coming out of the models or analysis coming out of the models, explaining what those results are and what they mean and how they’re getting generated. That becomes much more difficult as you start to lean more heavily on machine learning and AI-type methodologies,” Holden said.